SPARE Challenge
The Sparse-view Reconstruction Challenge for Four-dimensional Cone-beam CT
The SPARE challenge stands for Sparse-view Reconstruction Challenge for Four-dimensional Cone-beam CT (4D CBCT). The aim is to systematically investigate the efficacy of various algorithms for 4D CBCT reconstruction from a one minute scan.
What is the Rationale?
The goal of the SPARE challenge is to explore the possibility of high quality 4D CBCT while sparing patients the additional scan time and imaging dose that is currently used in the clinics.
4D CBCT allows the verification of tumor motion for thoracic patients immediately before every radiotherapy session. Currently a clinical 4D CBCT scan takes 2-4 minutes, as compared to one minute for a 3D CBCT scan. The recent emergence of image reconstruction algorithms that account for data sparsity offers new possibility for 4D CBCT reconstruction using a shorter scan. Specifically, the ability to reconstruct 4D CBCT images from a one minute scan would reduce scan time and imaging dose. Additionally, every radiotherapy centre would gain access to 4D CBCT using existing 3D CBCT scanning protocol.
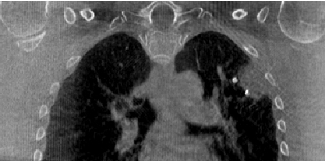
Current 4D CBCT from a one-minute scan.
The goal of the SPARE challenge:
high quality 4D CBCT from a one-minute scan.
The Challenge
Participants received CBCT projection data either acquired clinically or simulated. Prior CT data and training sets were also be provided. Using these datasets, participants applied their reconstruction algorithms and submitted the final reconstructions to the host within a timeframe of three months. The participants were allowed to apply any processing and algorithms to reconstruct the 4D CBCT images, including scatter correction, projection smoothing, iterative correction, etc.
Together with the reconstruction submission, the participants were asked to include descriptions of the methods they applied. The host evaluated the performance of each algorithm using a set of ground truths that were not available to the participants. The best performing algorithms in terms of image quality and accuracy were identified.
Host
The SPARE challenge was led by Dr Andy Shieh and Prof Paul Keall at the ACRF Image X Institute, a research institute under The University of Sydney. Collaborators who have contributed to the datasets included A/Prof Xun Jia, Miss Yesenia Gonzalez, and Mr Bin Li from the University of Texas Southwestern Medical Center, and Dr Simon Rit from the Creatis Medical Imaging Research Center.
Challenge Datasets
There are three main datasets in this challenge:
- Monte Carlo dataset
This dataset contains projections simulated from real patient 4D CTs, which are the ground truths for evaluating the reconstructed images. A total of 12 patients and 32 scans are included. There are three different types of simulated projections: no scatter, scatter, and low dose. This allows the comparisons of both reconstruction algorithms and scatter correction. - Clinical Varian dataset
This dataset contains oversampled clinical CBCT projections acquired from a Varian system using half-fan geometry. A total of 5 patients and 30 scans are included. The 4D CBCT reconstructed from the oversampled projection sets are used as the ground truths. The participants received the down-sampled projection sets. - Clinical Elekta dataset
This dataset contains oversampled clinical CBCT projections acquired from an Elekta system using full-fan geometry. A total of 6 patients and 24 scans are included. Similarly to the clinical Varian dataset, reconstructions from the oversampled projection sets are used as the ground truths, while the participants received the down-sampled projection sets.
For each CBCT scan, the participants received both the raw projection data and a 4D CT scan to be used as a prior. In addition, one or more training cases will be provided, where both the raw projection data and the ground truth reconstructions were available.
Timeline
December 2017 – Registration for participation opened.
15th January 2018 – Registration closed.
End of January 2018 – Participants received challenge datasets.
30 April 2018 – The deadline for submitting reconstruction results.
August 2018 – The results of the challenge study were presented at the AAPM Annual Meeting.
December 2018 – the challenge datasets were made publicly available for future research studies; the manuscript began being being prepared.
Winners
A total of 20 teams participated in the challenge, with 8 from the United States, 7 from Asia, 3 from Europe, 1 from Australia, and 1 from Russia. Iterative, motion-compensated, prior deforming, and machine learning techniques were adopted by 19, 8, 3, and 2 of the teams, respectively.
In the end, only 5 teams completed the challenge within the given time frame. The rest of the teams did not submit reconstruction for every scan in the simulated dataset. As the simulated dataset stood at the core of this study, the results are only presented for the five teams that completed the challenge. The five teams and their methods are, in no particular order:
- MC-FDK: the motion-compensated FDK [1] implemented by Dr Simon Rit from the CREATIS laboratory. A prior DVF is built from the pre-treatment 4D-C. Using this DVF, a FDK reconstruction is performed but with the backprojected traces deformed to correct for respiratory motion.
- MA-ROOSTER: the motion-aware spatial and temporal regularization reconstruction [2] implemented by Dr Cyril Mory from the CREATIS laboratory. The reconstruction is solved iteratively by enforcing spatial smoothness as well as temporal smoothness along a warped trajectory according to the prior DVF built from the pre-treatment 4D-CT.
- MoCo: the data-driven motion-compensated method [3] implemented by Dr Matthew Riblett from the Virginia Commonwealth University and Prof Geoffrey Hugo from the Washington University. The motion-compensation DVF is built using groupwise deformable image registration of a preliminary 4D-CBCT reconstruction computed by the PICCS method.
- MC-PICCS: the motion-compensated (MC) prior image constrained compressed sensing (PICCS) reconstruction [4] implemented by Dr Chun-Chien Shieh from the University of Sydney. The reconstruction is solved using a modified PICCS algorithm, where the prior image is selected to be the MC-FDK reconstruction.
- Prior deforming: the reconstruction is solved by deforming the pre-treatment 4D-CT to match with the CBCT projections [5]. This method was implemented by Dr Yawei Zhang and A/Prof Lei Ren at the Duke University.
- S. Rit, J. W. H. Wolthaus, M. van Herk, and J.-J. Sonke, On-the-fly motion-compensated cone-beam CT using an a priori model of the respiratory motion, Med Phys 36, 2283 (2009), ISSN 2473-4209, URL http://dx.doi.org/10.1118/1.3115691.
- C. Mory, G. Janssens, and S. Rit, Motion-aware temporal regularization for improved 4D cone-beam computed tomography, Phys Med Biol 61, 6856 (2016), ISSN 0031-9155.
- M. J. Riblett, G. E. Christensen, E. Weiss, and G. D. Hugo, Data-driven respiratory motion compensation for four-dimensional cone-beam computed tomography (4D-CBCT) using groupwise deformable registration, Med Phys 45, 4471(2018), https://aapm.onlinelibrary.wiley.com/doi/pdf/10.1002/mp.13133.
- C.-C. Shieh, V. Caillet, J. Booth, N. Hardcastle, C. Haddad, T. Eade, and P. Keall, 4D-CBCT reconstruction from a one minute scan, in Engineering and Physical Sciences in Medicine Conference (Hobart, Australia., 2017).
- L. Ren, J. Zhang, D. Thongphiew, D. J. Godfrey, Q. J. Wu, S.-M. Zhou, and F.-F. Yin, A novel digital tomosynthesis (DTS) reconstruction method using a deformation field map, Med Phys 35, 3110 (2008), ISSN 0094-2405, URL http://www.ncbi.nlm.nih.gov/pmc/articles/
PMC2809715/.
Download the challenge datasets
The SPARE Challenge datasets are now publicly available: Download the SPARE Challenge datasets
Files in the download link include the participant datasets (4D-CT prior and projection data), ground truth data (for evaluation), and MATLAB scripts to automatically quantify algorithm performance as conducted in the SPARE Challenge study. Instructions on how to use the datasets and the scripts are included. Anyone is welcome to use the SPARE Challenge datasets for their research. Any study that makes use of the SPARE Challenge dataset should cite the following reference.
Shieh CC, Jia X, Li B, Gonzalez Y, Rit S and Keall P. AAPM Grand Challenge: SPARE – Sparse-view Reconstruction Challenge for 4D Cone-beam CT. 2018 August. American Association of Physicists in Medicine Annual Meeting 2018. United States, Nashville.
Contact
Dr Andy Shieh: andy.shieh@sydney.edu.au